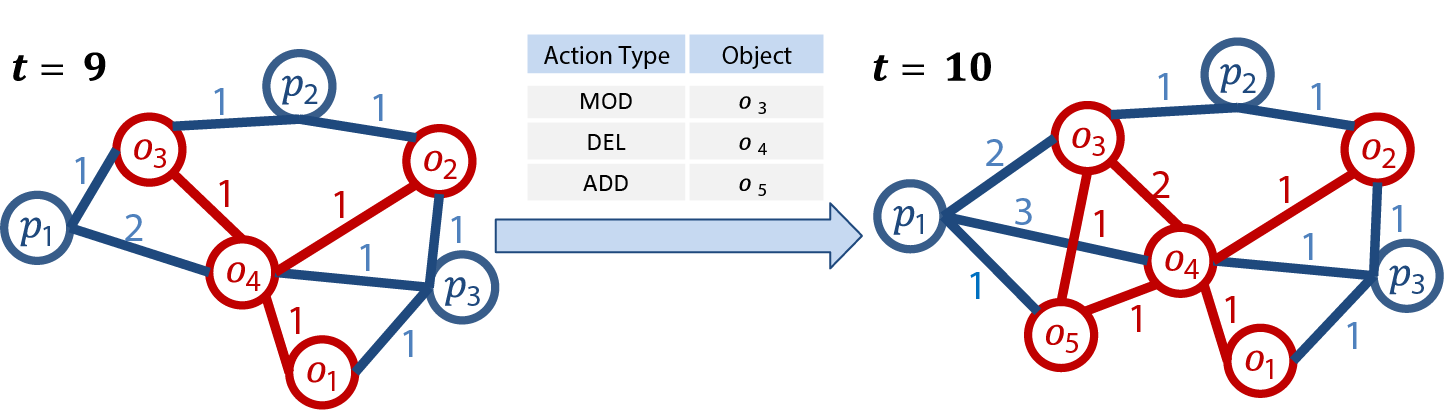
Complex tasks such as treating patients with chronic conditions and developing software products are typically accomplished by teams that collaborate over an extended time duration. To remain coordinated, team members need to be aware of others' activities if those activities are likely to affect their own actions. However, identifying such interactions and sharing information appropriately is challenging, especially when the activities of team members are loosely-coupled. In practice, team members often either lack important information about others' activities, or are overwhelmed by the need to review too much information. This paper presents Personalized Change Awareness, a new approach for supporting team coordination which aims to automatically identify and share the subset of information about others' activities that is most relevant to each of the team members. The paper formally defines the computational problem of information sharing in loosely-coupled teamwork, which underlies the personalized change awareness approach. It defines a new representation, Mutual Influence Potential Networks (MIP-Nets) and an algorithm, MIP-DOI, that uses this representation to determine the information that is most relevant to each team member. In contrast to existing information sharing algorithms in multi-agent teams, MIP-DOI does not assume the availability of a priori knowledge of a team's possible plans, because human teams rarely explicitly define detailed long-term plans in advance. We demonstrate the ability of MIP-DOI to identify relevant information using simulations of collaborative activities. We further evaluated the contribution of personalized change awareness to team performance in a controlled user study. To this end, we developed a personalized change awareness mechanism for collaborative writing, which used MIP-DOI to determine which changes to share with each author. This evaluation demonstrates that the Personalized Change Awareness approach resulted in higher productivity and lower perceived workload without any change in final quality compared to the currently prevalent approach of sharing all change information. Our results also demonstrate that merely reducing the amount of information shared with co-authors is not enough: sharing a random subset of changes resulted in significantly lower quality of work than sharing a personalized subset of changes.
Ofra Amir, Barbara J. Grosz, Krzysztof Z. Gajos, and Limor Gultchin. Personalized change awareness: Reducing information overload in loosely-coupled teamwork. Artificial Intelligence, 275:204 - 233, 2019.
BibTeX